When we engage with clients to help them identify where and how to leverage big data for business value, we frequently use the Big Data Business Model Maturity Index (BDBM). This helps organizations understand how effective they are at leveraging data and analytics to power their value creation processes.

Applying the BDBM can help an organization identify how it should enact changes to people, processes, and technologies to enable the creation of analytic insight that drives its top-level strategic initiatives. Organizations that adopt this approach can utilize advanced analytics to couple new sources of customer, product and operational data, optimizing key business processes and uncovering new monetization opportunities.
However from an IT perspective, what does this look like? The traditional data warehouse just can’t support these new data and analytic capabilities.
Well, the time is right for organizations to embrace a data lake as the data management platform for advanced analytics and predictive insight. A data lake not only provides a repository for the collection of all sorts of structured and unstructured data, both internal as well as external to the organization, but it also enables data science teams to self-provision an analytic sandbox where they can rapidly ingest new data sources, ascertain their value and uncover new, more accurate predictors of business performance.
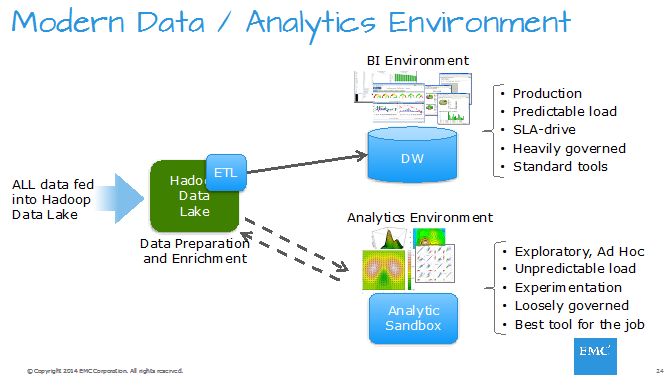
The data science team needs an environment where they can quickly test new data sources and analytics models without having to go through the laborious, multi-month data warehouse integration process.
And once the data is loaded into a data lake, think “load once and analyze multiple times” – across multiple analytic use cases.

The above chart maps the data sources – and the relative value of those data sources – to the analytic use cases in order to prioritize the data loading roadmap.
A data lake also provides a benefit to organizations that are looking to free up expensive data warehousing resources by offloading the ETL processes. This allows those processes to take advantage of the inexpensive, scale-out, natively parallel Hadoop environment.
And ultimately who knows how the data warehouse might be transformed as technologies such as HAWQ deliver more of the value of SQL and Business Intelligence (reports and dashboards) to the Hadoop data lake environment.
With these systems in place, organizations can efficiently store and analyze their data to surface the insights that help them monetize data opportunities. These advancements through the phases of the BDBM enable the metamorphosis into a truly data-driven business.
As more and more organizations embrace the data lake approach, I couldn’t be more excited to watch the results.